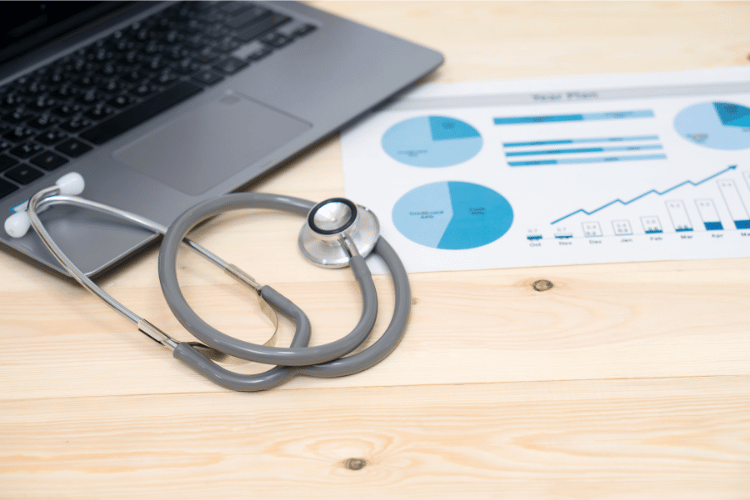
Due to increasing IT support, hospitals produce a large amount of digitally stored information. Therefore, the question arises more frequently as to what benefit the analysis and use of big data can offer.
By definition, big data in healthcare refers to electronic health records that are so large and complex that they are challenging to manage with conventional software and hardware, and they need to be more readily addressed with traditional or mainstream data warehouse tools and methods.
This definition is better than the usual 3V definition, as it clarifies that additional efforts are needed for existing solutions. The three V represents volume, velocity, and variety, which can be combined with the term “complex”, which points to another problem with the 3V definition, as complexity can manifest itself in very different properties from velocity.
Data for an extensive data system in hospitals include the following forms, in addition to the classic structured clinical and administrative data that are predominantly available in the hospital’s clinical information system:
· Omics data (For example, DNA or protein sequence data)
· Medical reference data from external sources (For example, clinical trial databases)
· Stream data from software on technical devices (For example, MRI machines or medical health apps)
· Unstructured text data (For example, physician and nursing reports)
· Environmental data (For example, on events, disease trends, and weather)
Linking these data requires syntactic and semantic harmonization before they can be used in databases for further analysis. In medicine, ontologies and standards such as UMLS< SNOMED, DICOM and LOINC have been developed in the past for semantic harmonization. If the aim is to go beyond joint storage – including horizontal and vertical linking of data – linkage procedures must be provided at the value, record and ontology levels.
New Types of Storage Are Required for The Big Data Context
First and foremost, these include NoSQL architectures that allow many very different objects to be stored without rigid schema specifications. Representatives are, for example, Cassandra, SAP HANA, MongoDB and Virtuoso.
In addition to such databases, big data applications may also need customized file systems capable of storing several million files efficiently and redundantly. But also, compression methods and advanced data warehousing functionalities.
Apache Hadoop is a framework that claims to handle all of these areas. It uses the Hadoop Distributed File System (HDFS) as its file system, HBase as its database, MapReduce or direct acyclic graphs as its distributed computing principle, and Hive as its data warehouse.
Methods from the field of machine learning are particularly suitable for innovative analysis of such data. Well-known representatives are support vector machines, random forests, artificial neural networks and conditional random fields.
Use Case 1: Improving The Diagnosis of Breast Cancer
Many different subtypes characterize breast cancer, but their characteristics have not yet been studied in detail. Important oncological data for breast cancer are omics data (metabolites, proteins and genetic profiles), imaging data (CT, MRI, etc.) and clinical data such as tumor staging and laboratory data. To achieve improved diagnosis and treatment, all data sources need to be integrated into a big data diagnostic pipeline and lead to a powerful diagnostic mechanism. The issues of real-time analysis, in-memory processing, parallelism, and required long-term memory must be clarified, especially for omics data. No machine learning method has been validated in clinical studies, so conventional statistical analyses should be used for the relevant analyses.
Use Case 2: Creating A Database for Cancer Research
The integrated application of big data can lead to answers to more complex questions and hypotheses in the field of oncology. First, medical oncology researchers are interested in integrating as much knowledge as possible, including from external data sources, into the research infrastructure. In this case, time-critical aspects are relatively rare.
However, interpretations of results play a more significant role here, as oncology questions are related to identifying new patterns rather than the consistent use of data in a complex but well-known diagnostic process. In particular, this means that the involvement of methodologists and visualizations is significant. Focusing on new hypotheses also implies that far more data and preliminary results may be stored than seems appropriate for economic reasons.
Use Case 3: Business Metrics
Hospital management is more interested in an overall strategy that also leads to a financial ideal than individual improvement opportunities. A possible question might be: what combination of DRGs at what volumes leads to optimal financial results for the hospital? Evaluating the appropriate strategy for a hospital includes, for example, reported hospital utilization, staff satisfaction or supplier relationships (with wholesale pharmacies, drug or food distributors, procurements, etc.). Optimization of the reference population may also be of interest. Usually, such analyses require only a clinical data warehouse with OLAP (online analytical processing) functions. In this respect, this case serves as an example to clarify that only some uses of digital data should fall within the realm of considerable data processing efforts.
A significant area of concern with big data is the quality and validity of the results. As large volumes of data can no longer be manually assessed for reliability and quality alone, established and automated procedures are required to determine the data quality. Additional issues related to data protection and ethical issues associated with the use of data, such as personal and medical data. The more data collected on patients, the greater the risk of misuse, even if declared anonymous. However, it isn’t easy to anonymize large-scale data. Therefore, the data value chain needs additional organizational and technical safeguards.
Examples of metrics are developing clearly defined policies, consensus on implementing these policies, handling clear and punitive “terms of use”, monitoring all activities related to the data in question, accessing the data only through dedicated computers and using multi-layer firewalls.
The appropriateness of acquiring and developing big data technologies depends on adequately assessing the expected results and the risks involved. However, the network of healthcare providers will increasingly drive data collection that will make the use of big data inevitable
The Final Word
Big data is the future of healthcare. Thanks to the vast amounts of patient data generated by electronic medical records, wearable devices, genomics and diagnostic imaging, big data has become a critical tool for improving patient outcomes, reducing healthcare costs and advancing medical research.